J911: Quick Jammer Detection & Localization via Cell-Phone Crowdsourcing
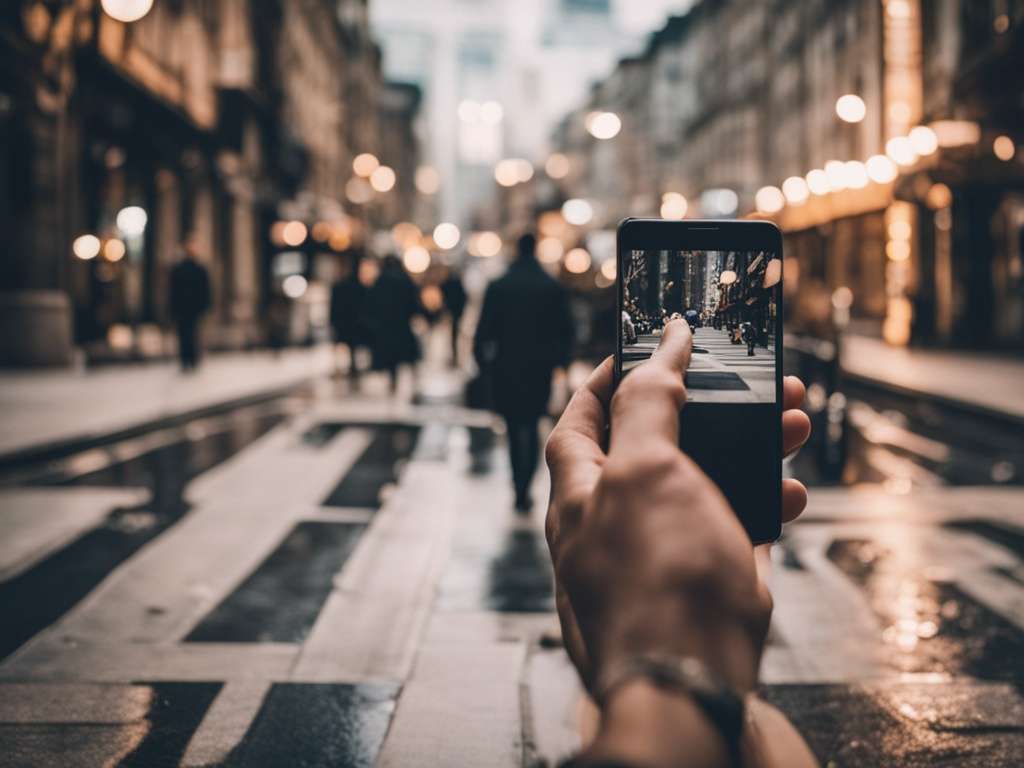
GPS jammers, easily obtainable yet costly, pose significant threats to safety, national infrastructure, and industrial revenue streams. To counter this, cell phones could integrate GPS jam-to-noise (J/N) ratio detectors. These detectors offer timely interference detection and effective localization, thanks to a flexible and updatable system where the crowd processing function resides in software.
- Affordable GPS Jammer: Just $33, Where to Find?
- Could Cell Phones Incorporate GPS Jam-to-Noise Ratio Detectors for Timely Interference Detection? Exploring the Viability, Locating Jammers, and Implementation Costs of Such a System.
- Could Cell Phones Incorporate GPS Jam-to-Noise Ratio Detectors for Timely Interference Detection? Exploring the Viability, Locating Jammers, and Implementing a Nationwide System.
- How Does J911 System Architecture Work?
- Is J911 System Performance Optimal?
- How to Detect a Signal Jammer?
- How to Locate a Signal Jammer?
- How to Position the J911 Effectively?
- Key Findings Revealed?
- Is the Affordable $33 GPS Jammer Widely Accessible?
- Is a Cheap $33 GPS Jammer Widely Accessible?
- Affordable GPS Jammer: Is $33 Too Good to Be True?
Affordable GPS Jammer: Just $33, Where to Find?
In early 2010, Newark Liberty International Airport experienced outages in its GPS Ground Based Augmentation System (GBAS), revealing a significant vulnerability in civil GPS infrastructure. These sporadic disruptions, caused by radio-frequency (RF) interference from unknown sources, spanned several weeks and affected the airport’s precision approach services. Investigators quickly identified nearby freeway vehicles as the likely perpetrators and embarked on a mission to apprehend the offenders.
Through a combination of cutting-edge interference detection technology and surveillance cameras, authorities successfully identified and apprehended the culprit: a truck driver in possession of a cheaply available $33 GPS jammer. This device, easily obtainable online, emits 200 mW of power and plugs directly into a vehicle’s cigarette lighter. The discovery of this affordable and accessible jamming device underscores the potential risks posed by such technology.
In response to this incident, the Federal Aviation Administration (FAA) has taken measures to relocate the airport’s GBAS system to a more secure location, away from the freeway. This proactive step aims to prevent future incidents and ensure the reliability and safety of the airport’s GPS infrastructure.
The widespread desire for privacy, coupled with a general lack of awareness about the potential devastation caused by GPS jamming, is likely to fuel an increase in the use of GPS jamming and spoofing for both legal and illegal activities. Alarmingly, most jammers remain undetected, causing unexplained GPS outages. It was only due to the advanced technology of the FAA’s GBAS that the root cause of one such outage was identified as jamming. However, the process of jammer detection, localization, and enforcement is resource-intensive and time-consuming, taking several weeks to apprehend just one offender. This highlights the urgent need for more efficient and effective solutions to combat GPS jamming. It’s sobering to think that a $33 jammer could potentially disrupt critical flight operations up to 10 miles away, underscoring the gravity of this issue and the importance of swift action.
Could Cell Phones Incorporate GPS Jam-to-Noise Ratio Detectors for Timely Interference Detection? Exploring the Viability, Locating Jammers, and Implementation Costs of Such a System.
Could Cell Phones Incorporate GPS Jam-to-Noise Ratio Detectors for Timely Interference Detection? Exploring the Viability, Locating Jammers, and Implementing a Nationwide System.
In this article, we delve into the feasibility of integrating GPS jam-to-noise (J/N) ratio detectors into cell phones, as proposed by Phil Ward at the ION-GNSS 2010. Can such a system determine the location of jammers? What would it take to make this a reality? Our findings suggest that in urban and suburban settings, warnings of jamming can be issued within 10 seconds, pinpointing jammer locations to within 40 meters. Imagine the impact on mitigating jamming incidents through swift law enforcement responses. Potential perpetrators would be aware of the risks and consequences, leading to a significant reduction in such activities. Moreover, the cost of establishing this nationwide system is surprisingly manageable. It doesn’t require billions of dollars or decades of effort. Instead, it demands a national commitment akin to the phase II wireless E911 initiative. We envision an Initial Operational Capability (IOC) by 2015, with comprehensive nationwide coverage achieved by 2017.
How Does J911 System Architecture Work?
The J911 System Architecture is designed with a focus on optimizing the automatic gain control (AGC) loop, a critical component found in virtually all GPS receivers. This AGC process ensures that the analog-to-digital (A/D) converter receives appropriate signal levels. The main objective is to fine-tune the gain, denoted as GA, so that a specific percentage of the 2-bit A/D converter outputs correspond to large values of 3 and -3. Typically, in a Gaussian noise environment, the VT percentage is adjusted to 35% to maintain A/D conversion losses at approximately 0.5 dB. Additionally, the architecture accommodates variations such as the 1.5-bit A/D converter, where the zero threshold is not implemented and three possible values (-1, 0, and 1) are output. This converter experiences about 0.9 dB of conversion loss when the VT percentage is set to 40%, significantly simplifying correlator processing.
For interference detection purposes, the control voltage to the AGC amplifier serves as a key metric, measuring jammer-to-noise power (J/N). Typically, under unjammed conditions, the nominal input power to an L1 C/A receiver hovers around -110 dBm. This power level is predominantly attributed to naturally occurring thermal and amplifier noise. Notably, the C/A code signal at -130 dBm, being a factor of 100 weaker, exerts no influence on AGC operation. However, as interference begins to rise above the thermal noise floor, the AGC responds swiftly by decreasing gain GA. This adjustment ensures that the correct percentage of large outputs is maintained. Response times to changes in input power levels are impressively fast, often less than 1 millisecond, allowing pulse jamming characteristics to be accurately determined. With knowledge of the control characteristics of the AGC amplifier, specifically the parameters (??, α), the receiver can precisely calculate the change in J/N given V1.
Accurate J/N measurements are possible, but likely require adding a switchable input step attenuator in the down-conversion chain, especially when dealing with small jammers nearby that can cause front-end saturation. To obtain the quiescent value, receivers can short the antenna on power-up as part of a built-in test prior to operation, or maintain and refine a historical value during normal operations, albeit with caution as spoofers and jammers may attempt to manipulate history-based values. Alternatively, if the receiver knows the quiescent V1 associated with a thermal noise-only input, it can obtain J/N on an absolute scale. For instance, in a 1.7 MHz bandwidth, the thermal noise floor is about -110 dBm, and so a J/N of 60 dB corresponds to a jamming signal strength of -50 dBm. However, measuring J/N above this level can become problematic for a low-cost GPS front-end. In a further refinement, receivers can include additional comparators set at -1.2 VB and +1.2 VB to enhance performance.
Optimize your receiver’s jamming resistance with our CE jammer detection technology. By identifying constant envelope (CE) jamming, such as CW, swept CW, or Gold code types, your receiver can adapt its VT percentage to achieve several dB of extra protection. This innovative approach, developed by our team at Texas Instruments in 1986, enabled the TI-420 L1 C/A receiver to surpass P-code receivers in resisting CE jammers. What’s more, with minimal hardware upgrades, your L1 C/A receiver can not only measure J/N but also determine the approximate type of jamming it encounters, including pulse, constant envelope, and Gaussian. Imagine the possibilities: using this data to detect and locate jammers with precision. Consider the scenario in Figure 2, where a 200 mW jammer is positioned at the origin [0,0], and J/N (dB) is mapped based on its relative location. This visual representation offers valuable insights for enhancing your receiver’s performance and resilience against jamming threats. Discover the power of CE jammer detection and take your receiver’s capabilities to the next level.
The J911 system, similar to the E911 system, effectively utilizes existing infrastructure and standards to locate signal jammers. When a wireless E911 call is placed, it’s routed through a mobile switching center (MSC) where it’s identified as a 911 call. By aggregating data from phones reporting J/N ratios and their positions, we can pinpoint the jammer’s location. Phones closer to the jammer report higher J/N ratios, providing valuable insights. Additionally, information about phone types and physical orientations helps interpret and correct raw J/N data, enhancing accuracy. Leveraging this comprehensive approach, the J911 system ensures efficient jammer identification and localization.
Creating a federal J911 PSAP to process J/N measurements into jammer location estimates, using the E911 system as a basis, would not be overly problematic. This is due to the widespread distribution and established infrastructure of the E911 emergency response system in the United States. Currently, there are 6,149 PSAPs distributed across the country, designed to handle wireless emergency calls. These calls are typically connected to a specific PSAP based on the caller’s location, which is determined by the cellular carrier. Under Phase II requirements, E911 call takers receive both the caller’s wireless phone number and their location information. This level of integration and data sharing provides a solid foundation for the proposed J911 system. Furthermore, 95 percent of PSAPs already have some Phase II E911 capability, indicating a high level of readiness and compatibility with potential upgrades or modifications. Software upgrades to phones, base stations, MSCs, and other components are routine and often include new or modified message provisionss and capabilities, facilitating the smooth integration of the J911 system into the existing architecture.
Integrating jamming reporting into the existing infrastructure leverages message transport and routing facilities. The key addition is a processing facility for these reports, either federally or as an adjunct to PSAPs. While incorporating J/N measurement into phones is a straightforward hardware enhancement, modifying existing phones isn’t feasible. Fortunately, cell phones typically have a two-year lifecycle before replacement, accommodating the addition of jamming reporting capability through the normal replacement cycle.
Is J911 System Performance Optimal?
Optimize signal jammer location detection with curve fitting techniques. By analyzing J/N measurements from a crowd of randomly located cell phones, we can determine the jammer’s position. A grid of hypothetical jammer locations is created, and curve fitting is performed for each point. The location that provides the best fit is identified as the jammer’s position. This process is illustrated in Figure 3, assuming exact J/N and location measurements from the cell phones. In our example, a 200mW jammer is located at xy = [0,0], and 1,000 cell phones are uniformly distributed over a surrounding 1-square-kilometer area. A hypothetical jammer location grid with points 5 meters apart spans ±150 meters in x and y. At each hypothetical point, the 250 highest non-saturated J/N reports are used in a least-squares curve fitting process. This assumes that jamming strength falls off as 1/R^α, where α is typically in the range of 2 to 4 in the ground mobile environment. By optimizing this process, we can accurately pinpoint the jammer’s location, enhancing security and mitigating potential interference.
In practice, knowledge of cellphone locations is imperfect, and GPS will be unavailable for those phones near the jammer. However, there are alternatives for determining location. Specifically, J/N (dB) is presumed to be a linear function of log10(R), where R is the range from the reported observer position to the hypothetical jammer location. Cellular carriers utilize a plethora of location determination techniques based on round-trip timing between the cellphone and observing base stations. At each hypothetical jammer location point, the norm of the residuals is collected as a metric of how closely the jamming reports (J/N + location) matched the least squares curve fit. This metric, plotted in Figure 3, demonstrates that the best fit is obtained at the true jammer location. It is noteworthy that α = 2 aligns with a free space propagation model, further validating our approach. The smaller the norm of the residuals, the better the curve fit, indicating a higher degree of accuracy in pinpointing the jammer’s location.
The J911 system performance is influenced by several key factors, chief among them being the utilization of Wi-Fi-derived location technology. This method, which relies on visible access points (APs), has been commercialized by companies like Skyhook and Google, making it widely accessible in most regions. Typically, it offers positioning accuracies of around 30 meters, even in the absence of GPS. Furthermore, as many modern phones are now equipped with integral accelerometers, there’s potential for propagating position with good accuracy, even when GPS is unavailable. However, another crucial aspect to consider is the highly variable nature of J/N observations. There are three primary effects that contribute to this variability: errors in measuring J/N due to quiescent V1 errors, imperfect AGC amplifier characterization, and uncompensated receiver antenna gain directionality. Additionally, large-scale shadowing effects caused by buildings, hills, bridges, and other obstacles, as well as small-scale multipath effects, further contribute to the fluctuations in J/N.
To capture the complex effects of signal propagation, a log-normal model is employed, representing deviations from ideal free-space conditions. This model characterizes signal strength using median values, while Ï log-normal, expressed in dB, quantifies Gaussian random deviations from this median. Such models are instrumental in predicting statistical cellular coverage and exhibit a strong correlation with real-world observations. Figure 4 illustrates a jammer location metric computed using a similar process to Figure 3, but now incorporating observer location errors of Ïx = Ïy = 30 meters and Ï log-normal = 6 dB. Notably, even slight movements of the cellphone can significantly alter the Jamming-to-Noise ratio (J/N), as signals may traverse multiple paths, either constructively or destructively combining at the receiver.
In this scenario, the hypothetical signal jammer’s most precise position was determined to be at xyjammer = [10,45] meters, thanks to crowd consensus. Although individual cell phone measurements may have been of lower quality, collectively they provided a remarkably accurate jammer location estimate. It’s worth noting that these cell phones have Wi-Fi-based positioning, with performance goals being within ±6 dB of the free space value 68% of the time, and ±12 dB 95% of the time. These are considered moderate standards. Before proceeding further, let’s briefly touch on crowd size and cell phone densities. Assuming a 70% cellular penetration rate, we’ve outlined approximate cell phone densities for various suburban and urban areas in Table 1.
The J911 system exhibits remarkable performance, particularly in dense cellular environments. Consider a scenario with 1,000 cell phones per square kilometer, a common density in many urban areas. Figure 5 illustrates the jammer location accuracies achieved under these conditions, assuming a uniform distribution of devices. Based on 500 independent simulation runs, this figure plots jammer location radial error statistics when processing 25, 100, 500, or 1,000 measurements. The radial error, given by J-EQ, significantly improves as more measurements are incorporated. Processing data from the entire crowd yields radial errors of 14 meters or better in 50% of the trials and better than 27 meters in 90% of the trials. This raises the question: why not process the complete set of measurements obtained from the cell phones to optimize accuracy?
To manage traffic and minimize false alarms during a jamming event, the process is split into two key phases. First, the detection phase identifies the jamming occurrence. Then, the locating phase pinpoints its precise position. This approach ensures that the cellular infrastructure isn’t overwhelmed, even when multiple cell phones are observing and reporting the event.
How to Detect a Signal Jammer?
To minimize power consumption during standby, cell phones are assigned to specific page groups based on their unique IMEI. In GSM, there are 50 such groups. Phones wake up to listen to the PCH based on their group, checking for incoming calls. By adjusting jammer reporting based on the phone’s page group or IMEI, we can limit initial traffic surges. During the detection phase, the system also identifies the type of interference event, ensuring efficient operation. This method balances power use and call reception, vital for maintaining smooth cellular communication.
Identify solar events from jamming with ease. A flat J/N response across locations indicates a solar flare, not localized jamming. True jamming events show a distinct geographic center with high J/N in a specific area. Plus, CE interference, not Gaussian, is a clear sign of human-caused disruption, helping pinpoint the source. Stay informed, distinguish between natural and man-made interference, and locate the culprit with confidence.
How to Locate a Signal Jammer?
When interference is traced back to jamming, the next step is jammer localization. While it may seem intuitive to use phones closest to the jammer for localization, phones with saturated J/N meters are not ideal. Instead, non-saturated phones offer valuable RSSI data that correlates well with distance. These phones, especially those nearest to the jamming source with high J/N readings, tend to encounter fewer signal propagation issues. During a jamming incident, the J911 PSAP manages traffic by limiting reports to only those phones registering a J/N value exceeding J/Nmin. As Figure 5 illustrates, processing the complete data set enhances jammer location precision compared to using a reduced dataset.
Optimizing jammer localization techniques can significantly enhance accuracy and efficiency. By processing the entire crowd, we achieve radial errors of 14 meters or better in half of the trials and surpass 27 meters in 90% of the tests. However, solely relying on the top 250 strongest J/N values impacts jammer snapshot localization precision, resulting in radial errors of 47 meters or less in 50% of cases and over 110 meters in 90% of trials. The silver lining is that the cellular network traffic generated is reduced to just one-quarter. In other words, for a given traffic handling capacity, we can update jammer locations at four times the rate. Incorporating additional reporting criteria like page group membership, general location, or IMEI allows us to sample different cellphone populations at each snapshot interval. Furthermore, implementing a Kalman filtering approach to track and smooth jammer location estimates may ultimately yield better performance, especially considering that individual phones can move considerably over time.
Improving jammer localization accuracy remains a focal point of further exploration. One potential approach involves utilizing phones exhibiting saturated or elevated J/N indications for geographical centroiding. Moreover, integrating multiple methodologies could yield promising results. In scenarios where the jammer is identified within a moving vehicle, enhancing location precision becomes feasible by confining the hypothetical jammer position grid to roadways, leveraging map data as a reference. These avenues present opportunities for deeper investigation. Figure 6 extends the analysis from figure 5, exploring scenarios with significantly reduced cell-phone density. Across all scenarios, comprehensive data collection and processing remain essential. As expected, a higher number of observers leads to enhanced jammer localization accuracy. However, even in areas with lower cell-phone densities, the system performs admirably, achieving a 50-meter accuracy 50 percent of the time and a 100-meter accuracy 90 percent of the time, assuming 100 phones per square kilometer.
Improving jammer location accuracy amidst propagation variability and measurement errors is feasible, even in moderately populated areas. Our analysis, as illustrated in Figure 7, reveals radial accuracy statistics for various Ï lognormal values (4, 6, 8, and 10 dB). As expected, the reliability of J/N measurements deteriorates with increased propagation variability and/or cellphone measurement errors. Consequently, the accuracy of jammer location estimates also suffers, but not catastrophically. Similarly, simulations with larger cellphone location errors showed moderate performance losses in jammer location accuracy. Overall, Figures 5 through 7 highlight that crowd size and crowd selection algorithms, rather than the accuracies of individual measurements, are the main driving factors in jammer-location accuracy. These insights pave the way for more effective jammer detection and localization strategies, especially in densely populated areas where signal interference is a common challenge.
How to Position the J911 Effectively?
The wireless operators had little enthusiasm for implementing wireless E911 due to its substantial hardware requirements for mobile station (MS) position reporting, with cell phones being an example of MS. However, E911 now serves as the technical foundation for numerous revenue streams, most notably the location-based services (LBS) industry. GPS jamming poses a direct threat to this revenue stream, particularly as GPS becomes integrated with vehicle navigation systems and intelligent highway systems. In this evolving landscape, cellular carriers will play a pivotal role in providing the necessary communication facilities. GPS jamming, therefore, represents a significant obstacle to this future revenue potential. Additionally, cellular signal jamming poses a threat to national infrastructure and carrier revenue. Fortunately, the approaches described above are easily adaptable for detecting and locating cellular frequency band interference sources in a timely manner, addressing these emerging challenges.
The implementation of a J911 system presents significant potential benefits for cellular carriers, making it a compelling proposition for industry adoption. Drawing from the established wireless E911 framework, the realization of J911 can be achieved through a structured three-step approach. Initially, the process begins with Rulemaking, where the FCC, upon validating the necessity, would issue a Notice of Proposed Rulemaking (NPRM) outlining the system’s functional prerequisites. This would be followed by industry feedback, leading to the establishment of J911’s performance criteria and deployment timelines through an iterative process. This phase is estimated to span approximately two years. The next step involves Standards Setting, where established bodies from the wireless, LEC, and PSAP sectors would collaborate to formulate detailed standards for J911 implementation. This critical work would be primarily driven by industry representatives working together.
The implementation of J911 would seamlessly integrate into the existing cellular infrastructure, requiring no hardware modifications except for the MS portions. As part of the regular update and release cycle, J911 would be deployed, ensuring compliance with the FCC’s rulemaking and standards setting processes for new mobile stations. This standards setting process, encompassing various system components like MS and BSS standards, is estimated to take one to two years, enabling manufacturers to produce interoperable equipment. Consequently, over a two-year timeframe, mobile devices would gradually transition to J911-compatible models, marking the full establishment of the J911 system. Interestingly, this concept of widespread adoption and integration echoes the findings of Francis Galton’s 1907 experiment, where he observed the collective wisdom of a crowd at a county fair. Galton found that, despite vast differences in individual estimates and expertise, the average of all guesses accurately predicted the dressed weight of a fatted ox. Similarly, the phased rollout and adoption of J911 across the mobile ecosystem promises to harness the collective power of technology and standardization, ultimately resulting in a robust and interoperable system.
The crowd’s median estimate deviated just 0.8% from the actual value, highlighting their impressive accuracy.
Key Findings Revealed?
Creating a national infrastructure for detecting and locating GPS and cellular jammers is crucial. This capability would underpin rapid and effective enforcement actions. A crowdsourcing approach, utilizing a multitude of opportunistic cellphone-based observers, appears to be a plausible solution, providing timely and location-specific alerts. Although individual measurements may be of poor accuracy, the crowd consensus yields good accuracy. While this system may not reliably detect purpose-built precision power-controlled spoofers, it could detect coarser cellphone apps-style spoofers that might, for instance, be seen in road-use tax avoidance. However, numerous open issues remain. Jammer antenna gain patterns can adversely affect locating accuracy. To what extent can this be mitigated by mapping out antenna gain contours? How can multiple simultaneous jammers be resolved? Can map and propagation modeling-based aiding algorithms improve jammer location accuracy?
The proposed system allows for continual improvement even after deployment, as its crowd processing function is software-based. Although significant research is still required, this flexibility ensures the system remains open to enhancements.